Hello everyone and welcome back to the Cognixia podcast. Every week, we dig up a new topic from emerging digital technologies and share insights, ideas, information, stories, and more. We strive to inspire our listeners to learn new things and update their repertoire of skills to stay relevant and continue growing in their careers.
If you have been keeping up with the news, you might have heard that the Nobel Prize winners of 2024 were just announced recently. Interestingly, the Nobel Prize for Physics went to two pioneers of artificial intelligence – John Hopfield of Princeton University and Geoffrey Hinton of the University of Toronto, for foundational discoveries and inventions that enable machine learning with artificial neural networks. Why is this interesting, you ask? Well, physics and artificial intelligence aren’t exactly very deeply connected disciplines, are they? So, how does the Nobel Prize for Physics go to two pioneers in the field of artificial intelligence?
According to the Press Release from the Royal Swedish Academy of Sciences that gives out the Nobel prizes, “This year’s two Nobel laureates in Physics have used tools from physics to develop methods that are the foundation of today’s powerful machine learning. John Hopfield created an associative memory that can store & reconstruct images and other patterns in data. Geoffrey Hinton invented a method that can autonomously find properties in data, and so perform tasks such as identifying specific elements in pictures.”
That’s interesting but what exactly are these tools from physics that were used to further the horizons of machine learning and artificial intelligence?
Well, let’s take a step back for a bit and try to understand this. Generally, when we talk about artificial intelligence, we quite often mean machine learning using artificial neural networks. The inspiration for this technology was the human brain. The artificial neural network is inspired by the neural network in the human body – the nervous system. In the case of artificial neural networks the brain’s neurons are represented by nodes and they have different values. The nodes influence each other through the connections they have, which is like how synapses occur in our nervous system. These connections can get stronger and weaker. The neural network in AI must be trained to develop stronger connections between the nodes with simultaneously high values. Hopfield and Hinton have contributed significantly to artificial neural networks from the 1980s onwards.
Let us look at John Hopfield’s work in the field. John has invented a network that uses a method for saving and recreating patterns. The Hopfield Network, as it is named, utilizes physics that describes a material’s characteristics due to its atomic spin. This property of atoms makes them behave like a tiny magnet. The Hopfield network is described as equivalent to the energy in the spin system that we encounter in physics. The Network is trained by finding values for the connections between the nodes so that the saved images have low energy. If the Hopfield Network gets fed a distorted or incomplete image, then the network will continue working through the nodes and update its values so the network’s energy will fall consequently. So, the Hopfield Network works step-by-step to look for the saved image that is the closest to the incomplete or distorted one it has been fed.
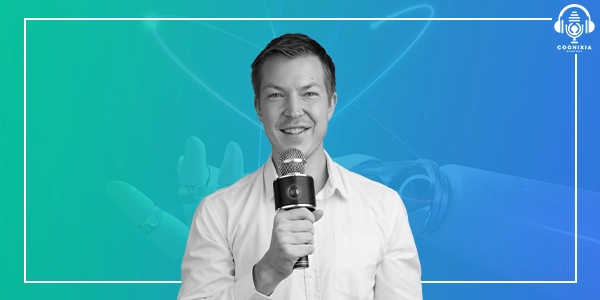
So, the principles and tools of atom spinning have been used to build the Hopfield Network.
Now, let’s look at the contribution of Geoffrey Hinton. Geoffrey built on the work of Hopfield by using the Hopfield Network as the foundation for a new network using a unique method called the Boltzmann Machine. This new network can learn to recognize the characteristic elements of any data type. So, for instance, it can learn to recognize the typical features of a particular type of image, say macro elements or folk art elements, or the peculiar features of a dog that can help identify its breed. For this, Geoffrey used tools from statistical physics and the science of systems built from many similar components. The resulting machine was then trained by feeding it examples that are very likely to be encountered by the machine in regular functioning. This Boltzmann Machine is very useful for classifying images or creating new examples of patterns on which the machine was trained. This development of the Boltzmann Machine has been critical and path-breaking in spearheading the AI evolution and has been indispensable for the current explosion of machine learning tools that we see all around us.
What is interesting is that artificial neural networks have been around since the 1940s. At that time, scientists – Warren McCulloch and Walter Pitts had first proposed the simple models of neural activity. The practical applications of artificial neural networks were practically negligible, limited at best, until the 1980s when Hopfield developed the Hopfield Network.
Tools from biophysics and statistical physics played important roles in making these developments possible, and this is why the Nobel Prize for Physics goes to these two amazing laureates. While mathematics and computer science have been significant for the development of the Hopfield Network and the Boltzmann Machine, it all boils down to the principles of physics, in essence. This has been a timeless truth that we have seen come to life time and again. Developments in one field or space trigger or inspire developments in another field, often even unrelated. It’s like a ripple effect. But today, as we talk about the Nobel Prize, we can join all these dots and present them to you, because, as Steve Jobs said, “You can’t connect dots looking forward, you can only connect them looking backward.”
So, the next time you ask ChatGPT or Gemini a question or use Dall-E to create an image for you or interact with an AI chatbot to get customer support, remember, it was physics that made it possible. Physics is a lot more than just speed, velocity, acceleration, semiconductors, resistance, force, etc. How technology will evolve further and how it will change the world as we know it, only time will tell.
For now, we congratulate John Hopfield and Geoffrey Hinton for winning the Nobel Prize for Physics and we wish them the best.
With that, we come to the end of this week’s episode of the Cognixia podcast. We will be back again next week with another interesting and exciting new episode. We hope we inspired you to keep your mind open and your skills sharp. See you again next week.
Until then, happy learning!